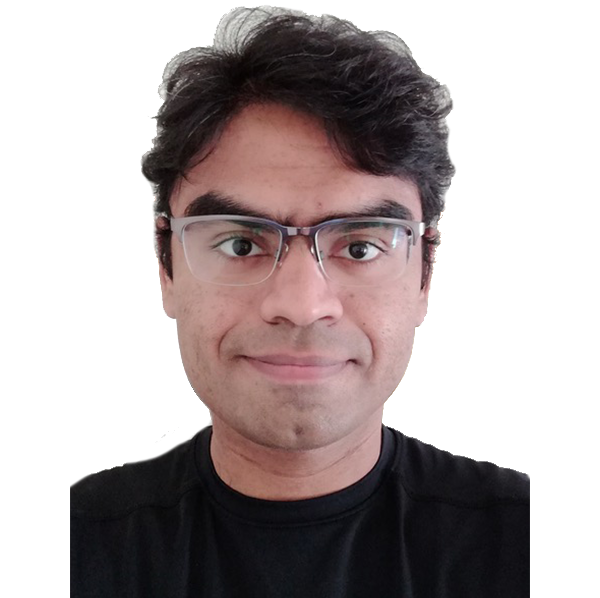
Shashank Subramanian
My research focuses on the development of an inversion framework for the patient-specific calibration of biophysical brain tumor growth models using clinical imaging data. My work aims at addressing two broad challenges. The first challenge is associated with insufficient temporal resolution in imaging data to drive image-based model calibration. I am working on developing biophysically-inspired regularization methods and inversion schemes to mitigate the severe ill-posedness of the model calibration inverse problem and enable reliable parameter estimates. The second challenge involves the high computational cost associated with 3D tumor growth simulation and parameter estimation. I am working on developing fast numerical solvers and efficient non-convex optimization algorithms that are integrated using scalable, parallel software capable of exploiting heterogeneous compute architectures (CPUs and GPUs). The ultimate goal is to utilize the estimated model parameters and spatiotemporal predictions within machine learning strategies to assist in predicting important clinical outcomes such as patient survival and tumor recurrence.
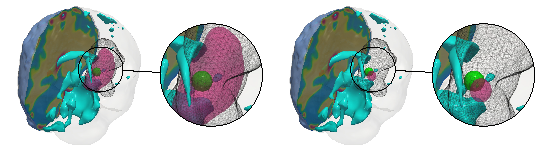
Reconstruction of tumor initial condition using two different regularization methods: (left) standard two-norm regularization and (right) physically-inspired sparse regularization. The images show a synthetically-generated brain tumor (gray wireframe), ground truth tumor initial condition (green volume), the reconstructed initial condition (magenta volume), as well as a section of the patient brain geometry. The two-norm regularized reconstruction (left) lacks localization capabilities and underestimates model parameters while the sparse reconstruction (right) is physically-consistent (sparse and localized) and yields significantly better parameter estimates (Subramanian et al. 2020, Inverse Problems).