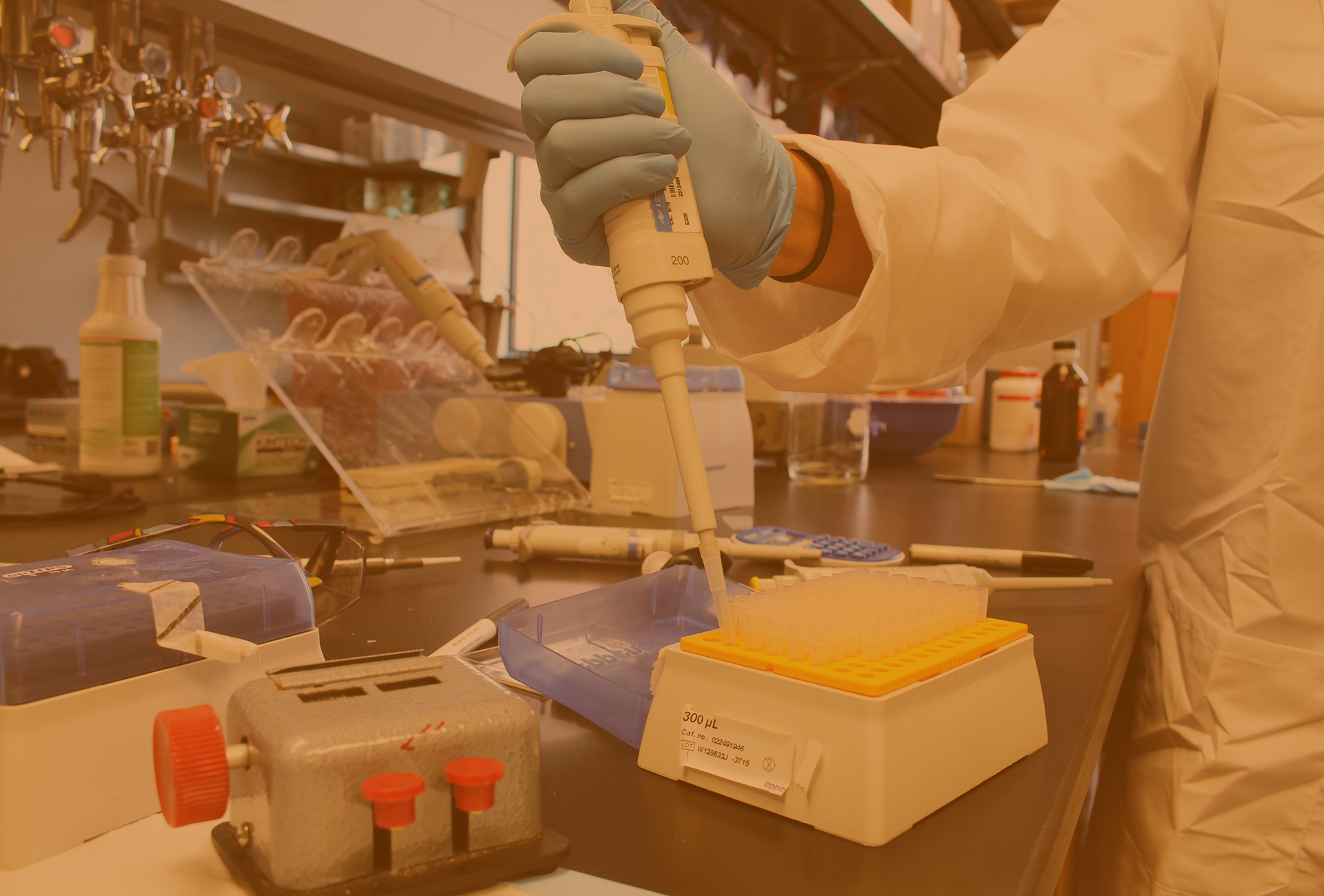
About
The Center for Computational Oncology
at the Oden Institute for Computational Engineering and Sciences
Mission
The past decade has witnessed a dramatic increase in our knowledge of cancer on multiple scales leading to a host of potential drug targets and subsequent clinical trials. Yet the outcome for many cancers has not improved. A fundamental reason for this sobering reality is that we do not have a validated theoretical framework to understand tumor initiation and development or how the individual patient may respond to particular therapeutic regimen; that is, there is no accepted mathematical description that enables us to generate testable, patient-specific hypotheses.
Currently, providing optimal therapies for a specific tumor phenotype, particularly with combinations of therapies, is extraordinarily difficult, as the number of potentially important adjustable parameters, such as the order and dosages of therapy, is too large to span in clinical trials and patient response is heterogeneous. Clinical trials too frequently lead to inconclusive and confusing results such that approximately half are never even published in the peer reviewed literature.
As our knowledge of cancer grows there is a desperate need to make real connections between those designing clinical trials and those studying mathematical models of tumor growth and treatment response so that the field of theoretical oncology can provide systematic, testable predictions of the response of individual patients to individual therapeutic regimens. The long-term goal of the CCO is to build a testable, mathematical theory of cancer. Cancer biologists could use such a theory to discover new biology, while oncologists could select the most promising treatment for an individual patient in a systematic fashion.
Approach
In our unique approach to tumor model construction, we require that all model inputs be constrained by experiment and/or measurable on the individual patient. Constructing models in this manner gives several distinct advantages over more common approaches based on population-derived metrics. Models naturally incorporate patient-to-patient heterogeneity and make quantitative, testable predictions of tumor progression on each individual tumor and patient, thereby allowing the model to be refined and/or verified.
Validated models predict the likely outcome of a therapy on individual patients and can be used to identify therapies most likely to succeed. In conjunction with patient monitoring, models can be used to evaluate the efficacy of the prescribed therapy early in the course of treatment, allowing discontinuation of futile therapy and substitution of more promising regimens. This will help to drive the shift from the traditional empirically developed methods of cancer biology and oncology to one in which prediction is the dominant paradigm, providing great benefit to the health and economic well-being of the nation. The University of Texas at Austin is well-positioned to be a leader in this effort.
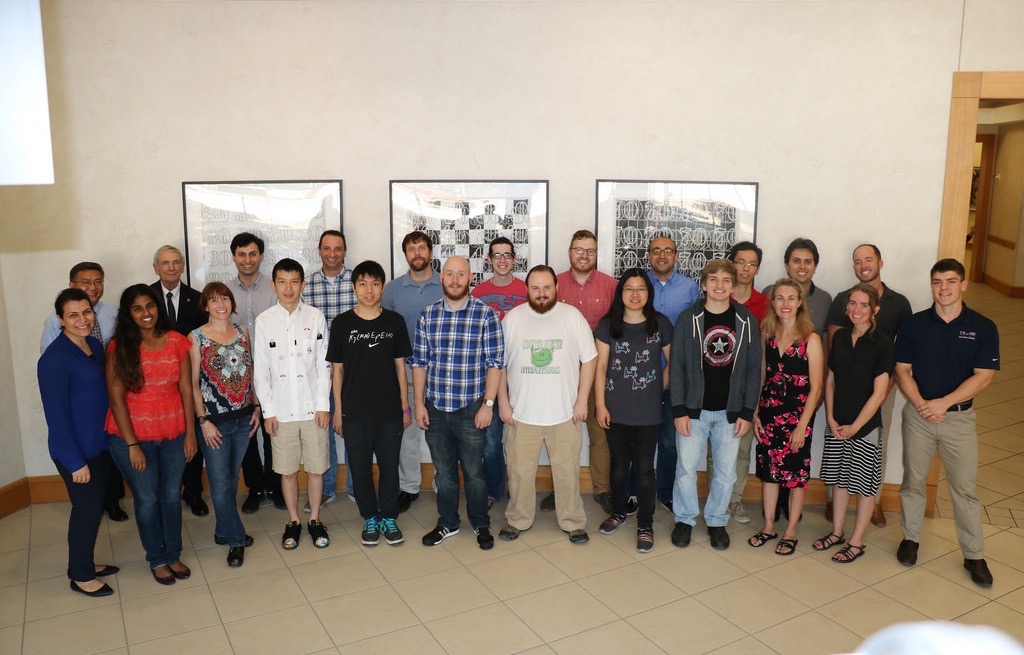

