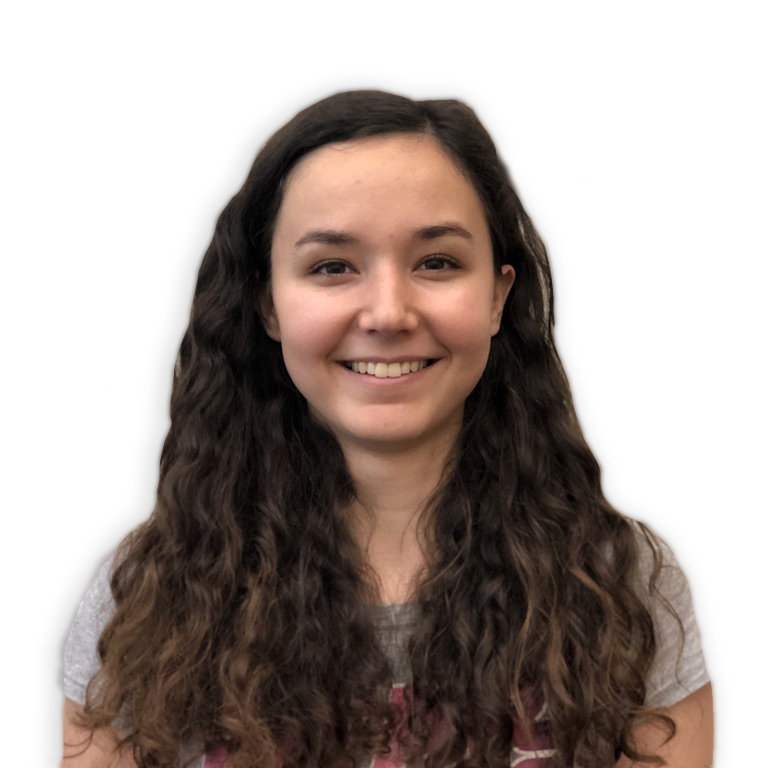
Kalina Slavkova
My research interests lie in the implementation of machine learning techniques to develop a dynamic contrast enhanced (DCE) MRI protocol that yields high spatio-temporal resolution images for quantitative imaging. Quantitative imaging provides diagnostically informative tissue properties related to the volume transfer constant (Ktrans) and extravascular/extracellular volume fraction (ve); however, this form of imaging is fundamentally limited by the resolution of the acquired DCE data. I am currently collaborating with a group in Electrical and Computer Engineering to devise a method for incorporating an image reconstruction algorithm – compressed sensing with deep image prior (CS-DIP) – to output high quality images from spatially undersampled data collected at an unprecedented temporal resolution.
Improving DCE MRI data resolution is also an important step in developing abbreviated breast MRI protocols for screening patients at high risk of breast cancer. Using nonlinear regression to fit various perfusion models to both clinical and simulated DCE data, I am working to determine the accuracy of estimating perfusion model parameters, such as Ktrans and ve, in tissues of interest using a subset of the timepoints in the original DCE time series. The overall goal of my work is to utilize CS-DIP to not only improve the usability of quantitative imaging in the clinical setting but to contribute to the development of abbreviated breast MRI scans that are significantly shorter but still diagnostically powerful.
(a) High spatial resolution frequency content data at the initial and final time points in a DCE MRI time series and spatially under-sampled intermediate data collected at a high acquisition rate.
(b) Central slice of a patient’s breast at 21.81 s from a DCE MRI scan with the region of interest (ROI) boxed in black.
(c) Ktrans parameter map for the ROI in panel (b) derived from a truncated version of the original DCE time series containing only 32 of the 66 original time points.
(d) Ktrans parameter map derived from the original DCE time series containing all 66 original time points.
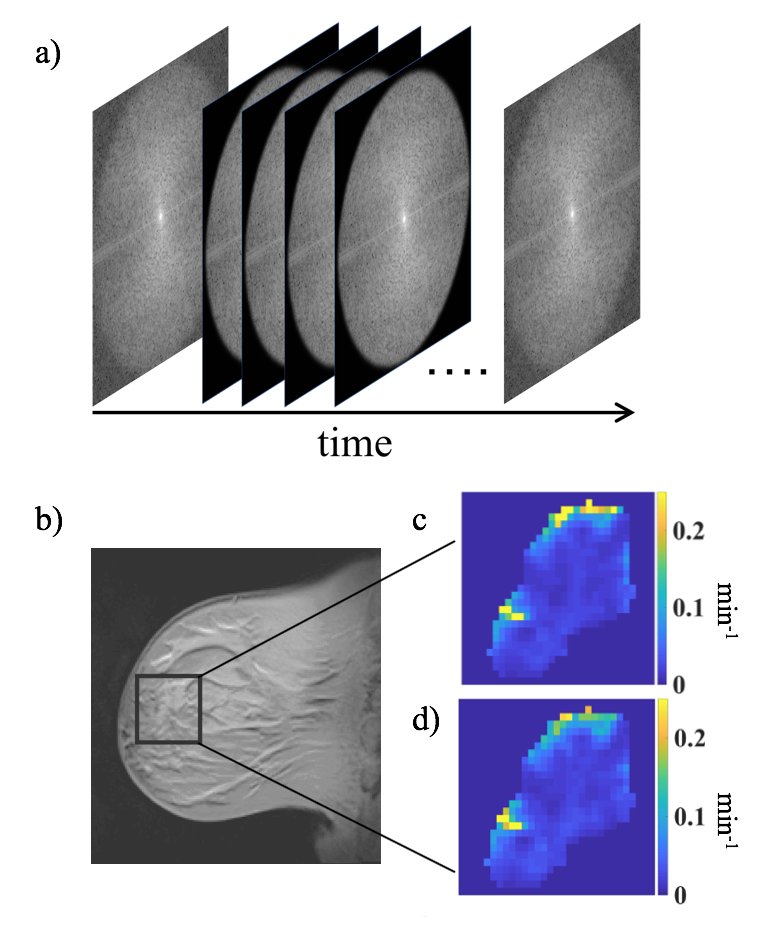