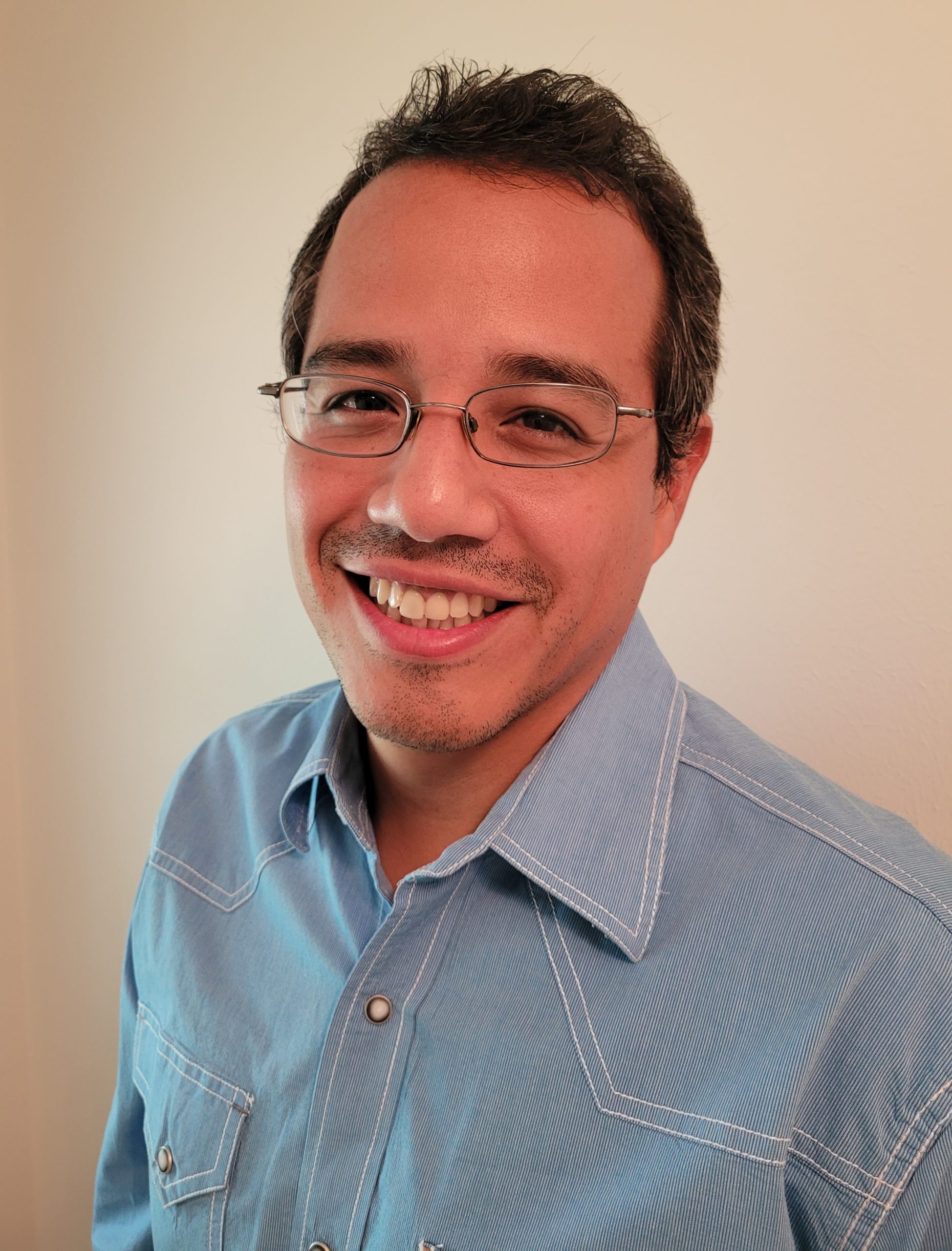
Edward Castillo
My research is focused on developing robust, automated, image processing & analysis algorithms for 1) detecting disease, 2) quantifying disease severity, and 3) predicting patient-specific treatment responses for both disease and healthy tissue. In addition to biomechanical modeling, this work requires many aspects of applied mathematics, including large scale, nonlinear, and nonconvex optimization, deep learning, iterative methods for large-scale linear systems, and parallel computing. For instance, my research team pioneered the development of Computed Tomography-Derived Functional Imaging (CT-FI). CT-FI is an image processing-based modality that employs mathematical modeling and scientific computing to generate quantifiable surrogates for pulmonary ventilation and perfusion from dynamic computed tomography (respiratory-gated non-contrast 4DCT). As a surrogate for ventilation, CT-FI uses image segmentation and registration to infer the local tissue volume changes induced by respiratory motion that are apparent on inhale/exhale lung CT image pairs. Using the same imaging data and processing, CT-FI quantifies variations in the spatial distribution of pulmonary blood mass as a surrogate for perfusion. My team and I have demonstrated that CT-FI has the ability to accurately quantify pulmonary function, quantify COPD disease severity, and indicate the presence of pulmonary embolism. However, the utility of CT-FI has been most established in the field of lung cancer radiotherapy.
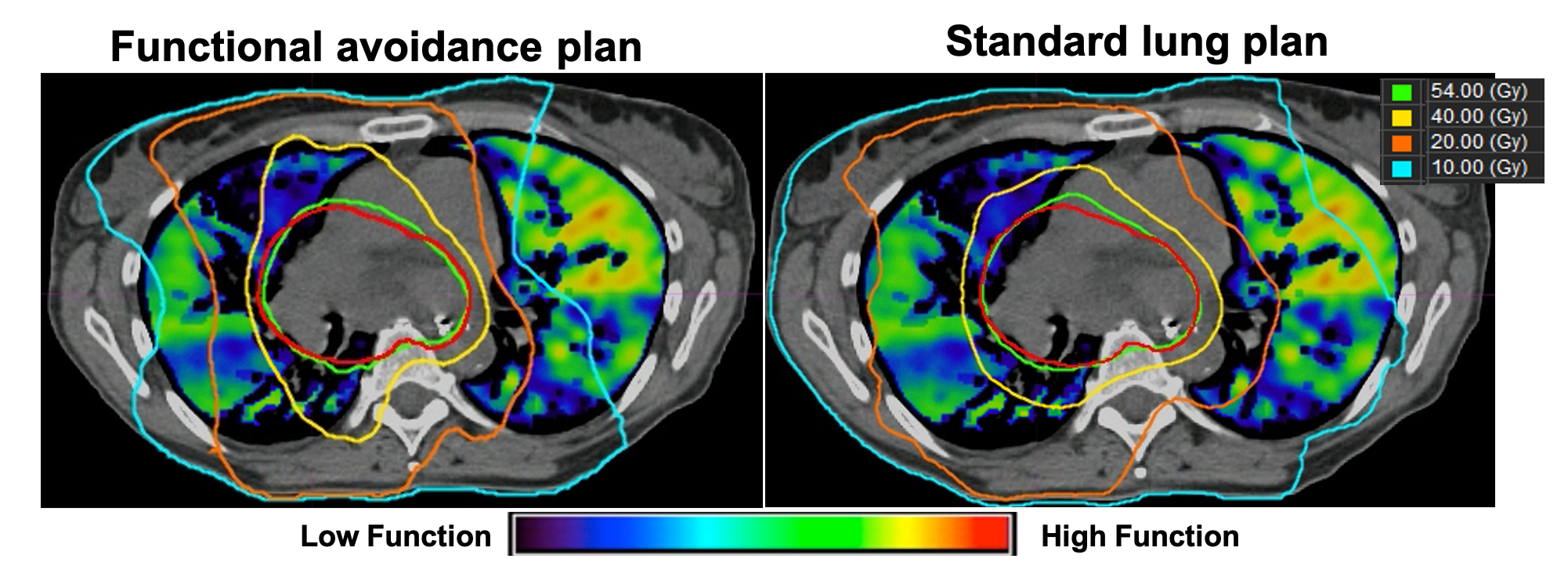